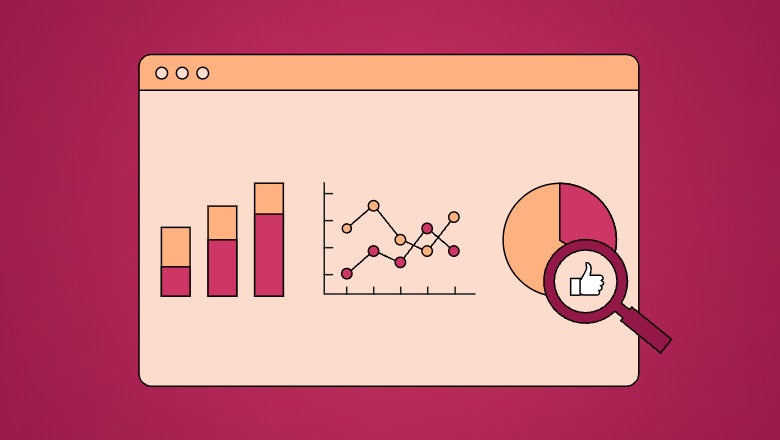
Social Media Analytics
Social media analytics: The complete guide
Starting a social media strategy? Learn how analytics play a crucial role in shaping effective marketing, understanding audience sentiment and achieving ROI through data-driven insights.
Reading time 8 minutes
Published on March 19, 2025
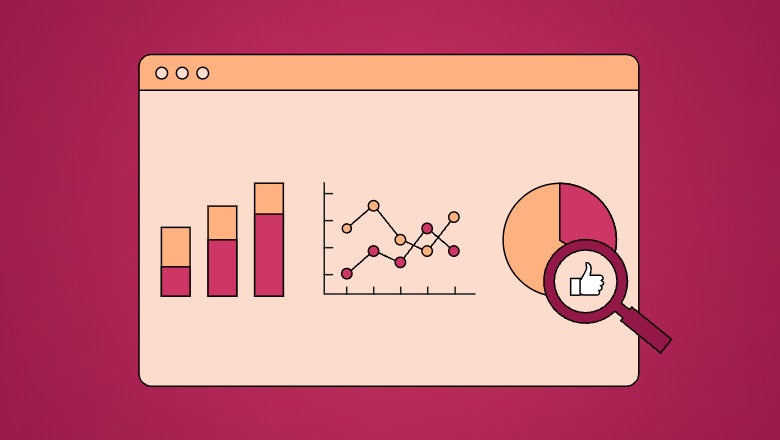
Table of Contents
Summary
- Social media analytics are essential for monitoring and assessing the success of marketing efforts.
- The key significance of tracking social analytics is to prove ROI, evaluate the success of social media campaigns and guide informed decisions for social media strategies.
- The main areas of social media analytics include performance metrics, audience demographics, competitor insights, paid ad results, influencer impact and brand sentiment.
When creating your social media strategy, you’re choosing goals and objectives that you want your marketing efforts to hit. But if you’re not monitoring your social media analytics, metrics and performance, how will you know if you’re hitting those goals?
Social media analytics are key to proving return on investment (ROI) and making informed business decisions. Luckily, nearly every social platform offers its own form of native analytics. Plus, there are a number of social media analytics tools that can help you monitor your performance in even more depth.
Throughout this article, we’ll touch on why social media analysis is so important, and the types of analytics you need to pay close attention to. We’ll cover which social media analytics tools you can use to help measure your success. We also made a video covering which social media analytics will help you dive deeper, so you can uncover insights for your marketing—and overarching business—strategies.
Social Media Analytics FAQs
How do I track my social media analytics?
There are tools built for social media tracking. You can read more about them in our post on the best social analytics tools, or you could try out Sprout Social’s Analytics tools for free today!
How do I report on social media analytics?
There is a slight nuance between social media analytics and social media reporting. While analytics refers to the data that you collect and analyze, reports are the documents you might put together to share internally.
Which social media metrics matter the most?
Not only is it important to have access to your social analytics, but you also need to know which metrics you should be tracking. That’s why we’ve written an article on the most important social media metrics. Start capturing data on your metrics and sharing them internally to show the work you’ve accomplished!
Additional resources for Social Media Analytics
The social media metrics to track in 2025 (and why)
12 of the best social media analytics tools for your brand in 2025
Social media analytics: The complete guide
6 skills social media analysts need in 2025 and beyond
7 AI tools for data analytics: A marketer’s guide
11 YouTube analytics tools to improve your marketing in 2025
Thanks for the memories: How to use the power of nostalgia marketing
The journey of a data point: Turning numbers into social media intelligence
YouTube analytics: How to analyze your YouTube data
LinkedIn analytics: The complete guide for tracking metrics in 2025
Reach vs. impressions vs. engagement: What’s the difference?
How to use Google Analytics for stronger social media reporting
How to set and achieve meaningful social media goals
Pinterest analytics: a guide for marketers
Social media SWOT analysis: what it is and how to do it
Share