How to use AI analytics for targeted business decisions
Regardless of size, most companies face the same challenges—tough competitors, and evolving customer and market demands. Add to these, workflow inefficiencies, data silos and rising costs, and there’s a growing need for tools that can help give you an edge. Enter AI analytics.
According to The 2023 State of Social Media Report, 96% of business leaders agree that AI and machine learning (ML) can help companies significantly improve decision-making. They’re eagerly adopting AI-enabled data for AI marketing to thrive and evolve in a data-driven and dynamic business environment.
In this article, we take a deep dive into AI analytics and how it differs from traditional data analysis methods for brand intelligence. We’ll also see how it can provide companies, like you, with sustainable market growth and an edge over your competitors.
Try a Demo of Sprout Social's AI and Automation Tools
What is AI analytics?
AI analytics is the process of extracting useful information from big data using advanced machine learning (ML) and AI tasks like natural language processing (NLP). It is used to identify patterns and trends in data for informed decision-making.
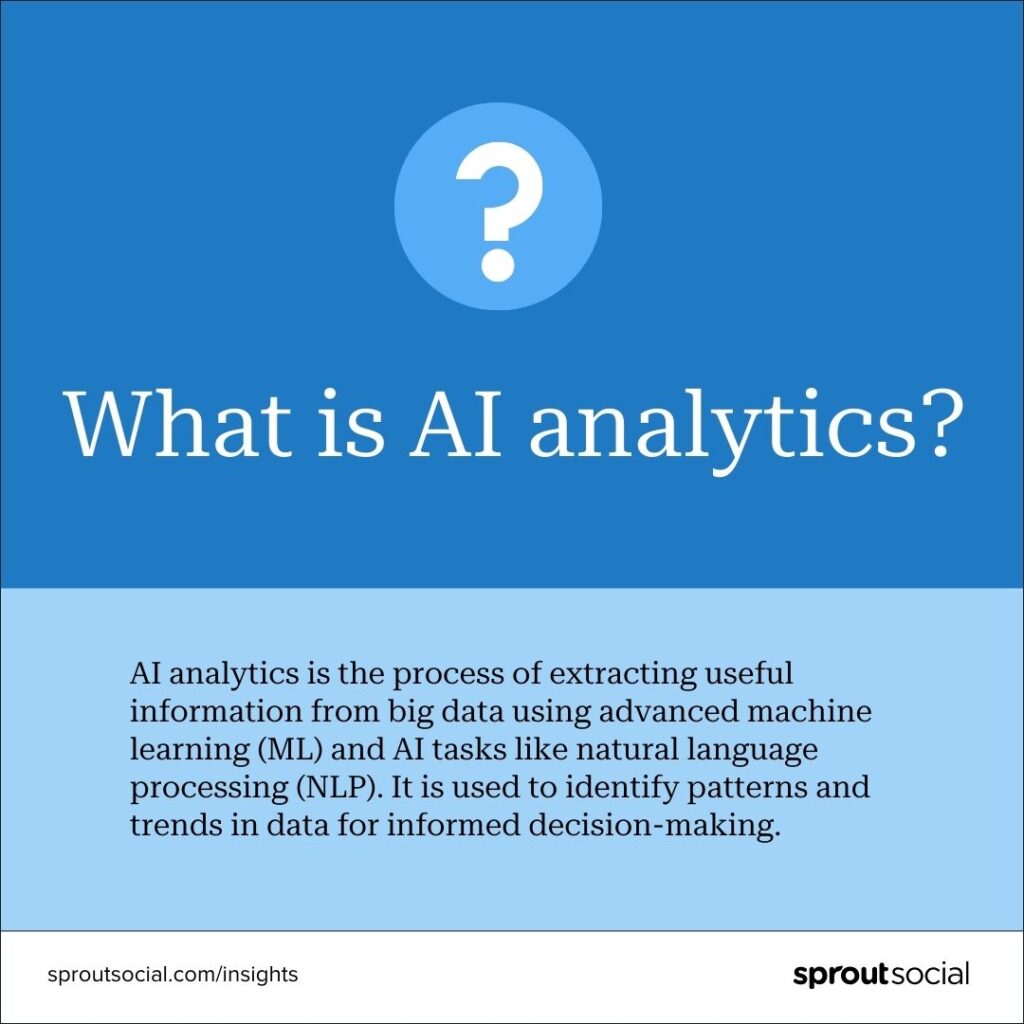
When applied in marketing, AI analytics extracts meaningful insights from various data sources such as social media listening, review websites, customer forums and survey data. These insights help cross-functional teams, such as marketing and sales, product research, human resources and PR, perform their jobs more effectively and efficiently.
And because data analysis is automated, it removes dependencies on manual tasks, increasing the accuracy of results and timeliness of business intelligence reports. It also frees up time so employees can focus on more strategic and complex parts of their jobs.
Bonus Resource: Get our top five AI social media marketing resources in one convenient toolkit. Download it for customizable templates and tips to drive smart AI adoption in your role and across your organization.
What is the difference between AI analytics and traditional analytics?
AI analytics uses machine learning algorithms to analyze big data, which enables it to process large volumes of complex, unstructured raw data quickly, accurately and easily. Traditional analytics, in contrast, requires more manual data analysis.
Even though traditional data analysis has been around for a long time, it has certain drawbacks that have led to the rising demand for AI analytics. For one, traditional analytics is not very effective in analyzing complex or large volumes of data.
More importantly, it can only analyze a limited scope of historical data to provide descriptive, diagnostic, predictive and prescriptive insights.
Here’s how AI analytics has transformed traditional analytics:
- Descriptive analytics: Interprets performance based on existing data and focuses on reporting a snapshot summary of the insights. AI analytics gives a more comprehensive report on continuous performance using trend analysis so brands have a holistic view of the various factors and changes over time.
- Diagnostic analytics: It is used to understand a business outcome by analyzing historical data for causation analysis. It identifies relationships in data to understand the root cause of an outcome. AI can analyze large volumes of historical and real-time data from disparate sources to find patterns, trends and anomalies quickly and more efficiently.
- Predictive analytics: Used to predict future trends and outcomes based on historical data using statistical models and forecasting techniques. AI uses machine learning and deep learning to predict future trends, providing faster, more accurate forecasts.
- Prescriptive analytics: Provides actionable insights from predictive analysis insights to improve business performance going forward. It is a combination of historical data and predictive variables, which can make the suggestions obsolete by the time you put them to use for a campaign. These AI insights enable you to analyze data in real-time by computing the change in variables as they happen so businesses can monitor and pivot campaign strategies promptly.
What are the four pillars of AI Analytics?
AI analytics depends on four critical AI sub-tasks to provide insights—machine learning, natural language processing, neural networks (NNs) and deep learning (DL). These technologies work together to extract meaningful information from raw and disparate data.
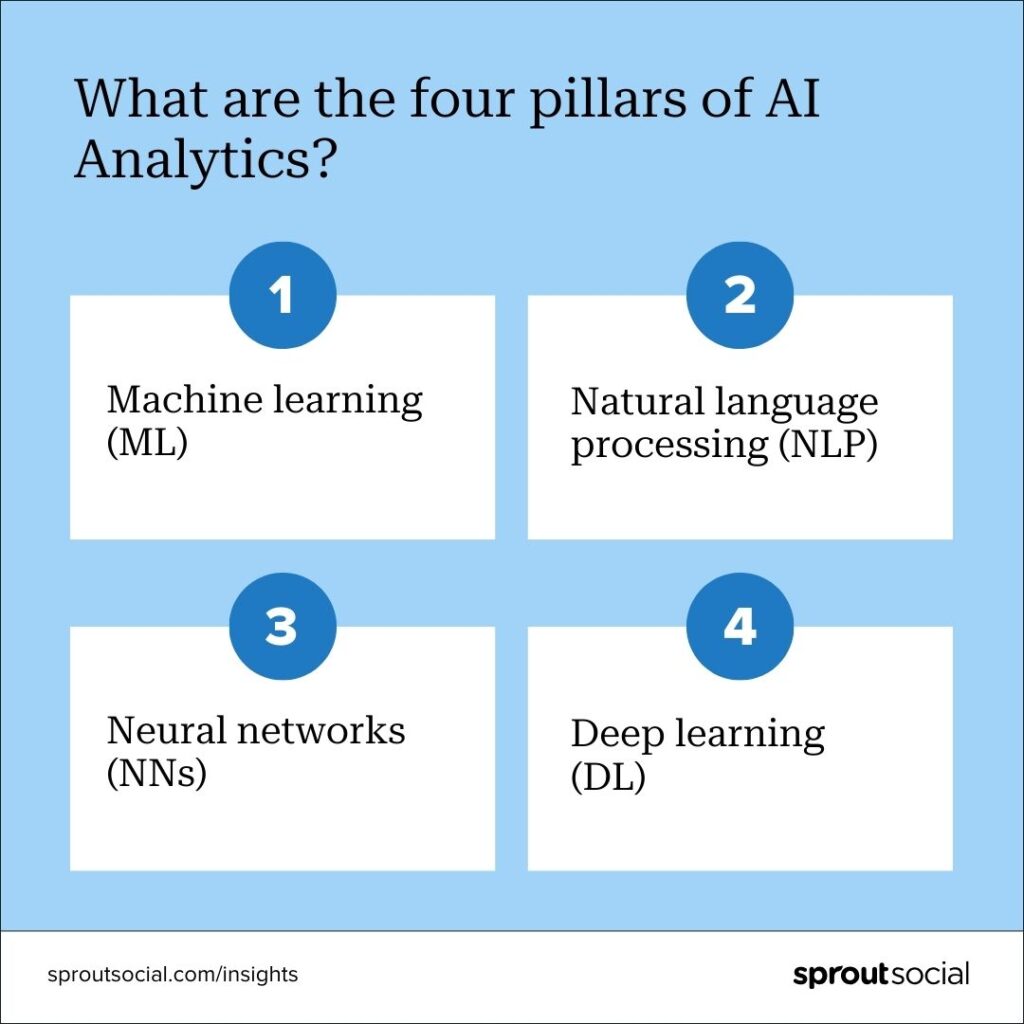
Machine learning
Machine learning helps AI analysis tools automatically identify data patterns so they can be synthesized for actionable insights. It assists in processing big data efficiently to identify relevant data points for predictive analysis.
This enables companies to, for instance, automate customer data analysis by processing customer experience feedback, product reviews and social listening data, in near real-time, to get timely insights.
Natural language processing
Natural language processing enables an AI analytics tool to understand and interpret human language so it can process and analyze it for meaningful information. NLP is pivotal to accurately analyzing social media listening data, customer reviews, blogs, news articles and other text-based data, including numbers and emojis.
Neural networks
Neural networks are algorithms built to understand relationships between data points identified by machine learning. They are inspired by how the human brain functions and comprise interconnected layers of nodes or neurons that remember relationships in data and learn from them. As a result, the AI tool progressively becomes smarter and more accurate with time, thus also making it cost-effective in the long run.
Deep learning
Deep learning algorithms are advanced NNs that process complex raw data using multiple layers of interconnected nodes. This enables it to adapt to a variety of data types and datasets and extract deeper insights for far more sophisticated analysis than traditional data analytics.
Why should we use AI for data analysis?
AI analytics empowers companies to automate repetitive tasks and process complex data more efficiently. Thus making scaling easy and frictionless. These tools can process thousands of API calls in minutes, giving you critical information about your brand, customer care and competitive analysis in a matter of minutes. This helps your team focus on more strategic aspects like providing stellar customer service and brand experience.
Sample this—per The 2023 Sprout Social Index ™, 76% of consumers notice and appreciate when companies prioritize customer support. Customers expect personalized service that’s more than just resolving an issue quickly. They want a personalized interaction that’s authentic and engaged.
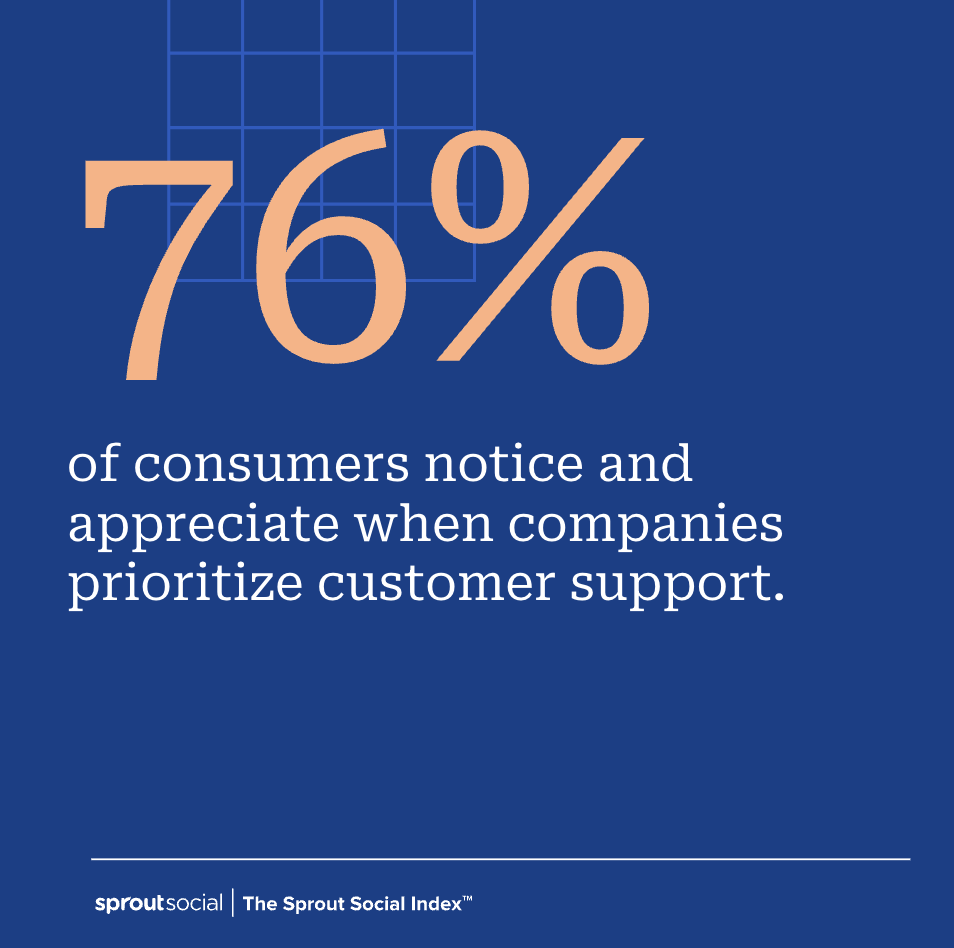
Achieving this level of personalization can only be done by having timely and accurate data at hand that will tell you how best to please consumers and nurture your loyal customer base.
For example, Sprout’s Listening capability will inform you of what topics are trending in social conversations about your brand, what related terms customers are using and the sentiment around those topics. You get a complete picture of what’s top-of-mind in social conversations and uncover common themes and topics regarding your brand, products and service.
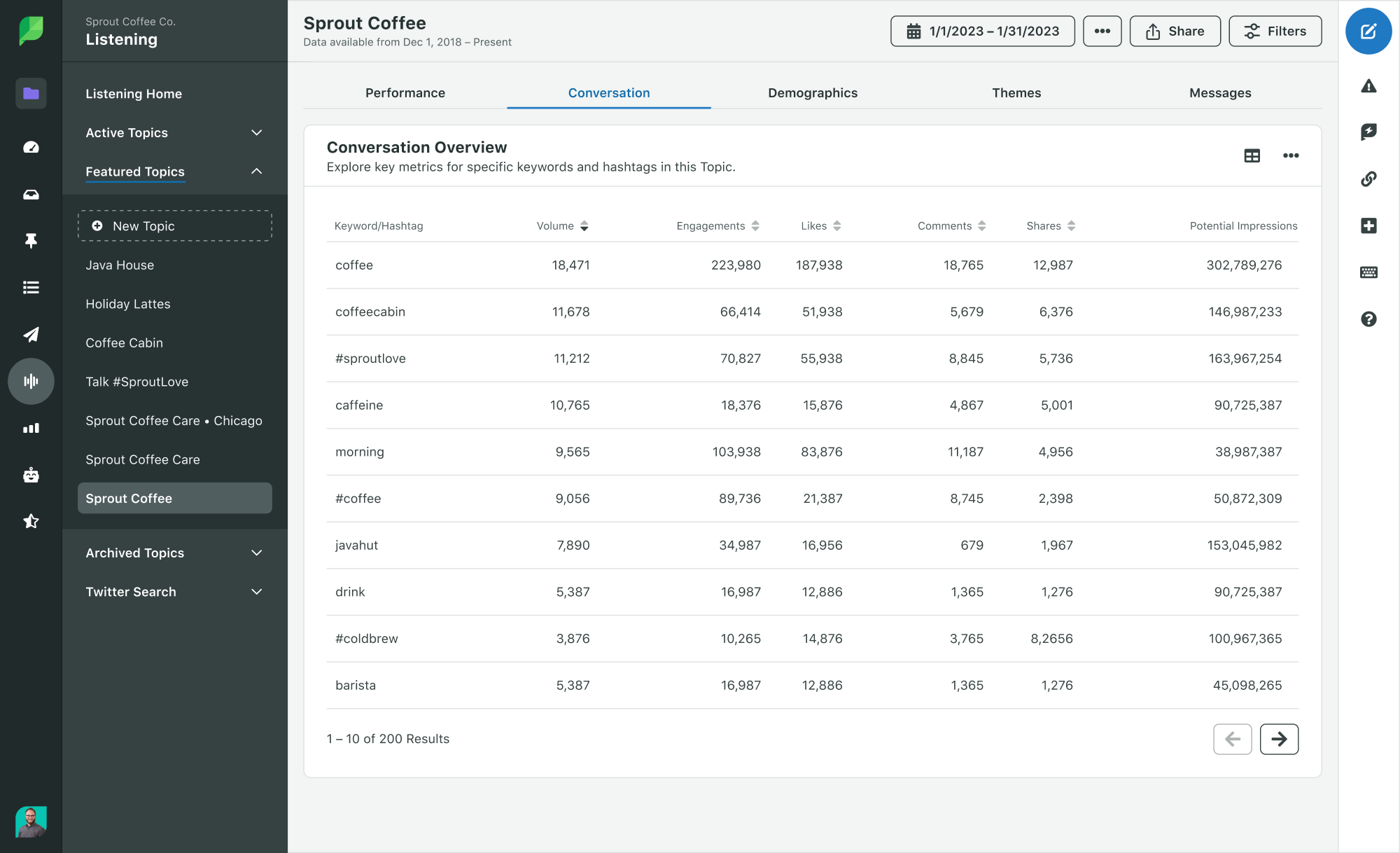
What are the benefits of applying AI analytics?
AI is transforming how businesses are operating by helping them automate tasks, so they can divert talent to areas that are more complex and strategic. Thus, enabling companies to grow and scale faster than before. Here are some key ways in which AI analytics is benefitting businesses today.
Automation
AI analysis helps businesses automate statistical calculations to monitor key performance indicator (KPI) metrics across platforms. This drives efficiency as time-consuming tasks are cut down and data analysis is expedited to derive relevant brand and customer insights. This ML-based automation gives back precious time to focus on planning and strategy while reducing workflow friction and workload.
For example, with an AI tool, companies no longer need to assign personnel to check every customer email or ticket to understand the request or complaint. These tools can seamlessly analyze all incoming messages and assign them sentiment so team members can quickly triage responses. In Sprout, also view spikes in brand mentions to keep a tab on brand sentiment and monitor potential crises.
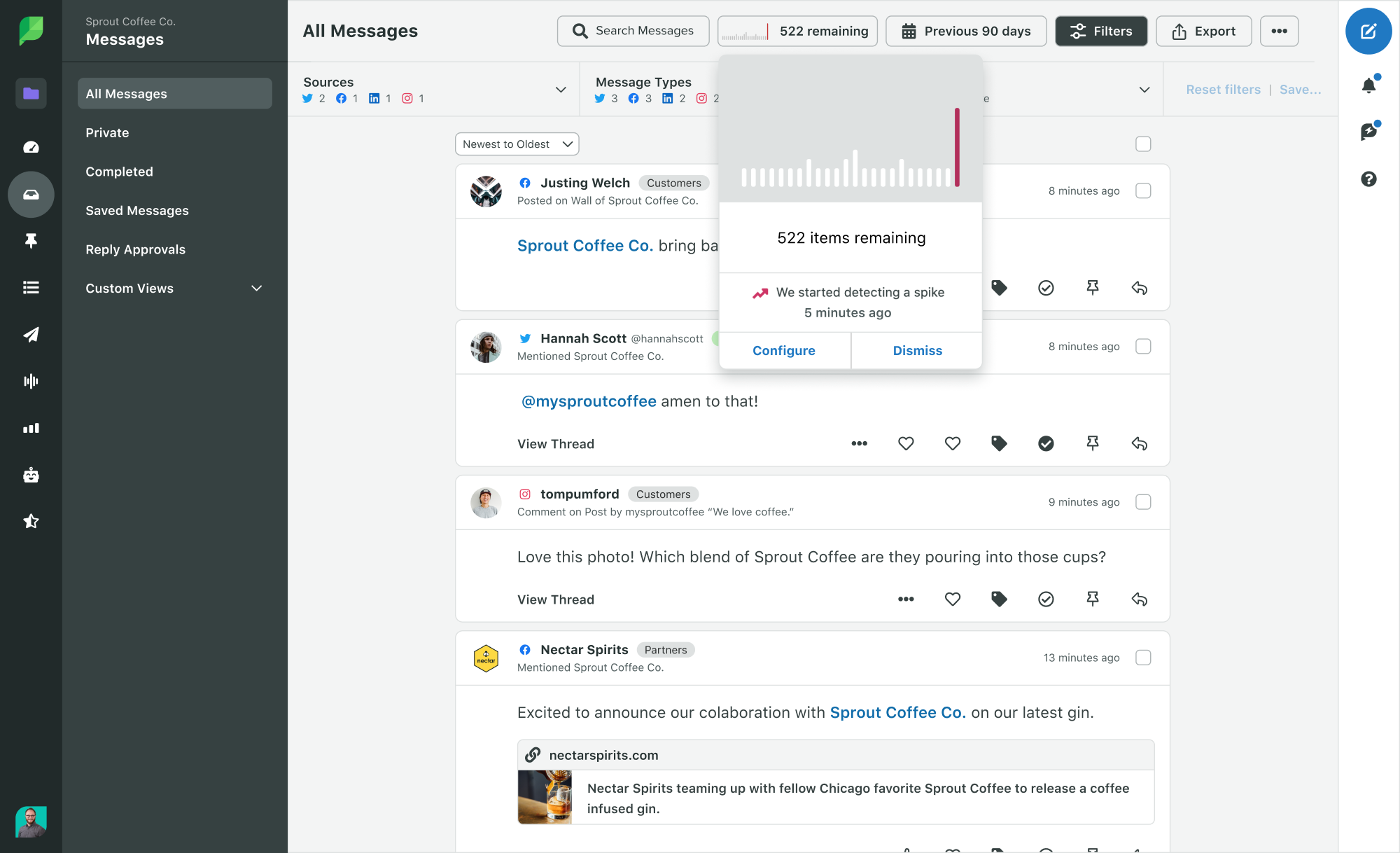
Scalability
AI analytics helps alleviate a company’s growth pains by efficiently handling large datasets from disparate sources to provide real-time insights—something not possible with traditional data analysis methods.
When integrated with a company’s customer relationship management (CRM) and business intelligence software, such tools exponentially increase the output of targeted insights by cross-referencing data points and identifying patterns in customer profiles and purchase patterns. Thus, giving a more holistic view of your current customers and prospects.
For example, Sprout’s Salesforce integration enables you to deliver exceptional customer experiences at every touchpoint by leveraging social data throughout the CRM. Create personalized touch points throughout the customer journey and increase the impact of your initiatives while implementing efficient workflows across the teams.
Accuracy
Results from AI data analysis are more accurate than manual data analysis methods. This is because there’s less scope for human error, biases or limitations such as excluding data subjectively, or while calculating complex metrics, especially from multiple sources.
Business applications of AI analytics
Companies use AI analytics to derive a stronger return on investment (ROI) from their social and digital data, while championing productivity and growth through intelligent automation. Let’s dig deeper.
Sentiment analysis
Sentiment analysis algorithms scan feedback data to prioritize key themes and topics, giving brands an in-depth view of common complaints or recurring product or service issues. In this way, brands can develop proactive strategies to ensure better customer experience to boost brand loyalty.
Sprout’s sentiment analysis tool gives you a snapshot of your brand sentiment by highlighting positive, negative and neutral sentiment in messages and social listening data. You can see sentiment trends over periods and analyze themes and conversations based on topics effortlessly. Use this data to improvise your product messaging, enhance customer care interactions and refine marketing strategies.
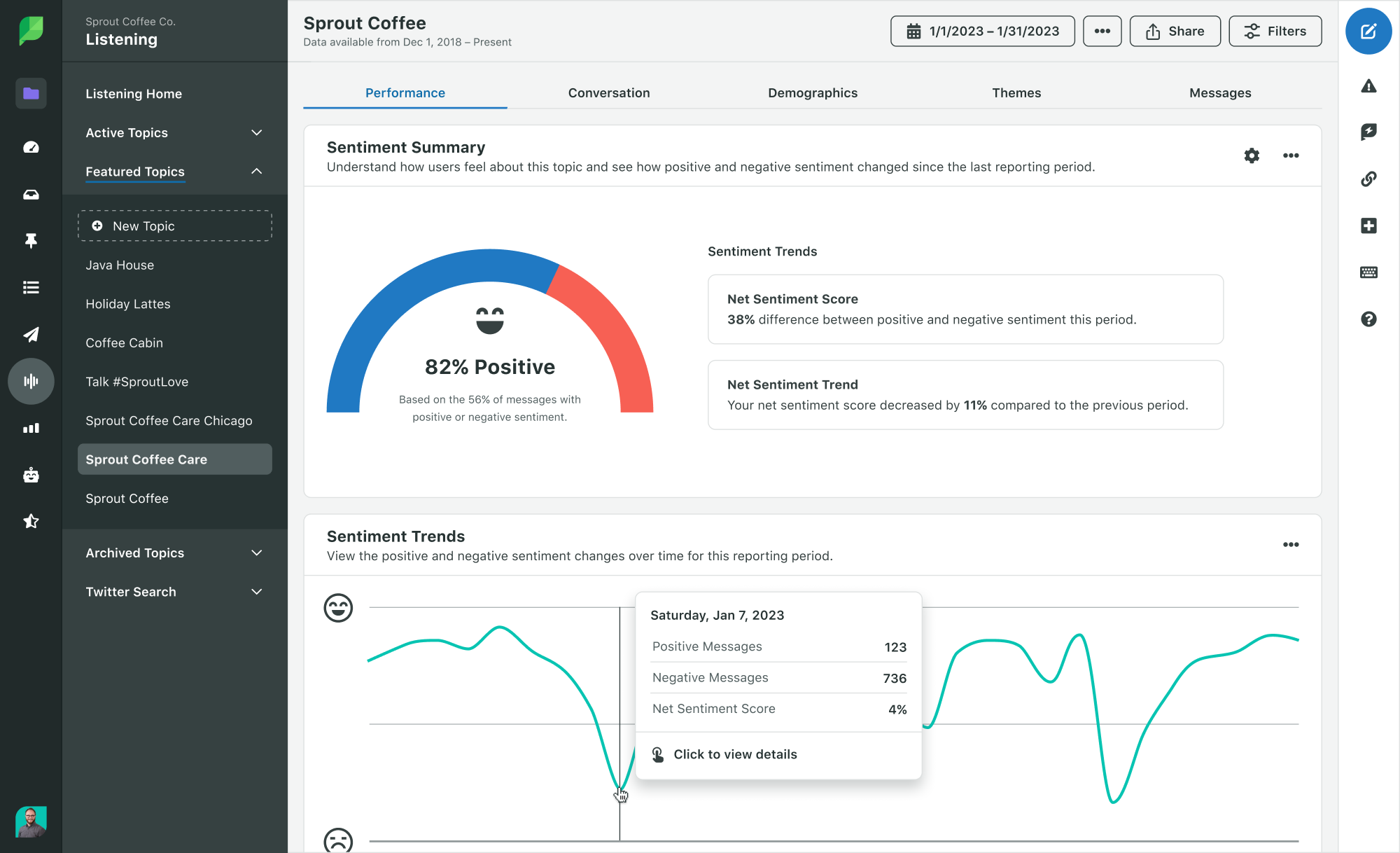
Demand forecasting
AI analytics enables companies to better understand customer interactions and purchase motivations. It helps them analyze large datasets quickly and efficiently to understand individual preferences and behavior. This helps a brand predict future demand based on specific customer demographics and market indicators. It also helps them enhance their offerings by customizing them specifically for different customer segments.
Sample this. A hotel, anticipating a surge in guests during the summer months, wants to provide a higher degree of personalized guest experience to achieve greater customer satisfaction. The company can get valuable insights in this regard by using an AI analytics tool to analyze customer messages, customer care emails, reviews and guest profiles. Through this, they may discover that most guests would appreciate childcare facilities so they can enjoy their stay even more.
By adding this facility during the summer months, the hotel is not only prepared for the increased demand but also leverages the opportunity to improve customer satisfaction, and thus stronger loyalty.
Business monitoring
AI analytics can analyze data in news articles, forums like Reddit and social conversations simultaneously, on both your brand and competitors. You can use this data to discover gaps in brand perception and take remedial steps for reputation management or to improve products or services.
In Sprout, you can also track competitor performance on different channels. For example, you can analyze your competitors on Facebook by getting in-depth metrics on KPIs such as public engagement average, fans average, audience growth and other metrics.
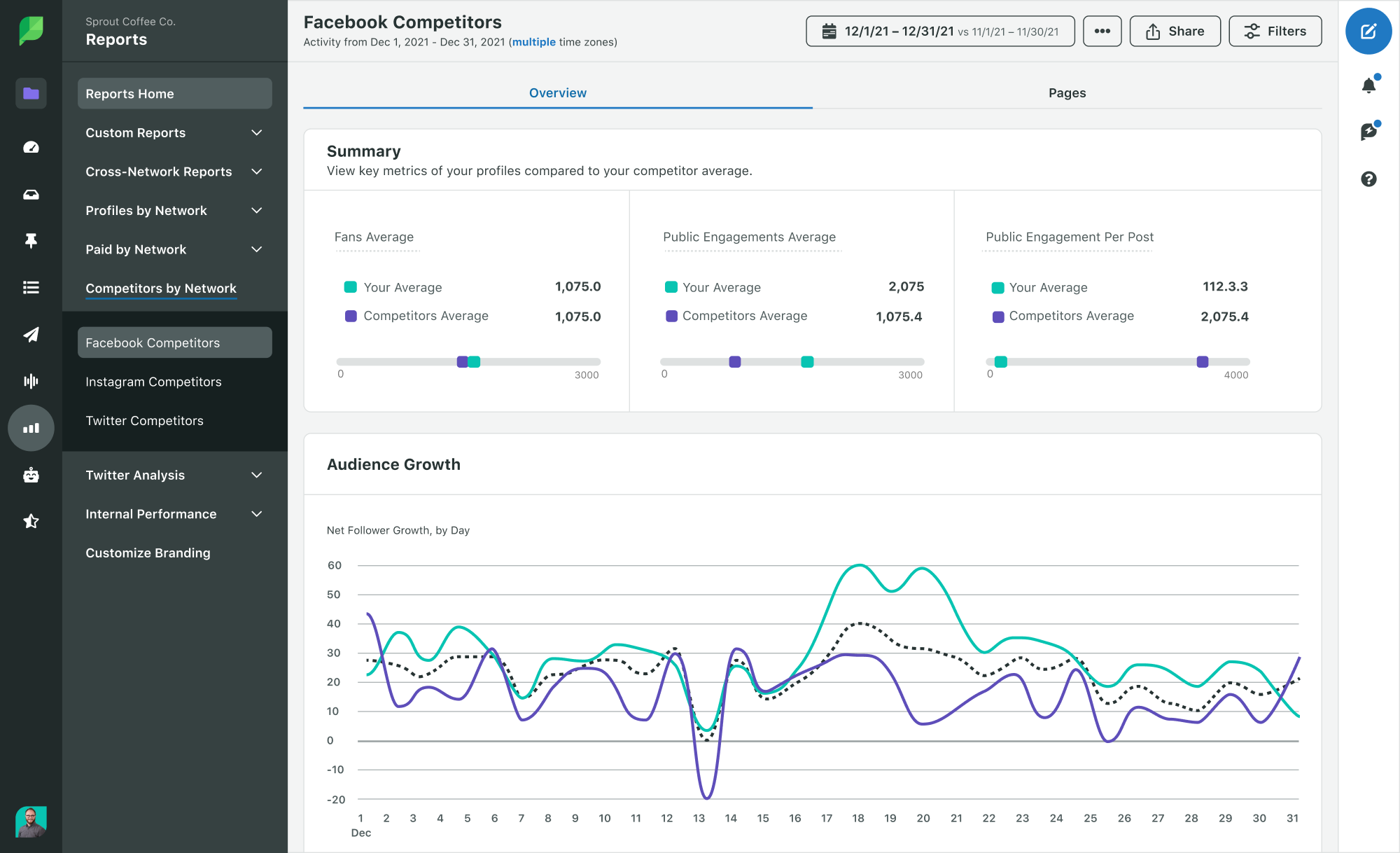
Top 5 AI analytics tools for your business
AI analytics tools offer varying degrees of details and insights. That’s why you must choose one based on your specific needs and characteristics, such as your industry, company size and target audience. You’ll also need to consider if the AI tool will seamlessly integrate into your existing IT infrastructure and workflows, and whether it will fulfill your scaling needs.
Here’s a list of industry-leading AI analytics tools that can provide you with the kind of insights you need to grow your business.
1. Sprout Social
Sprout Social is an all-in-one social media management solution that uses AI analytics for social media management, marketing, customer care and competitive intelligence. It also integrates with a variety of digital data sources including review sites like Reddit, CRMs like Salesforce and business intelligence tools like Tableau.
Our powerful social listening tool analyzes more than 600 million messages a day, identifying key topics to give you targeted insights. Analyze social conversations and customer messages to find topics that resonate the most with your audience and use those insights to inform your content and customer care strategy.
Conduct B2C and B2B market research effortlessly and strengthen your competitive strategy by using Sprout’s competitive analysis feature. Find impactful insights from key metrics like topic summaries, audience demographics and total engagements to inform your decisions and conduct a market-gap analysis to find new avenues for growth.
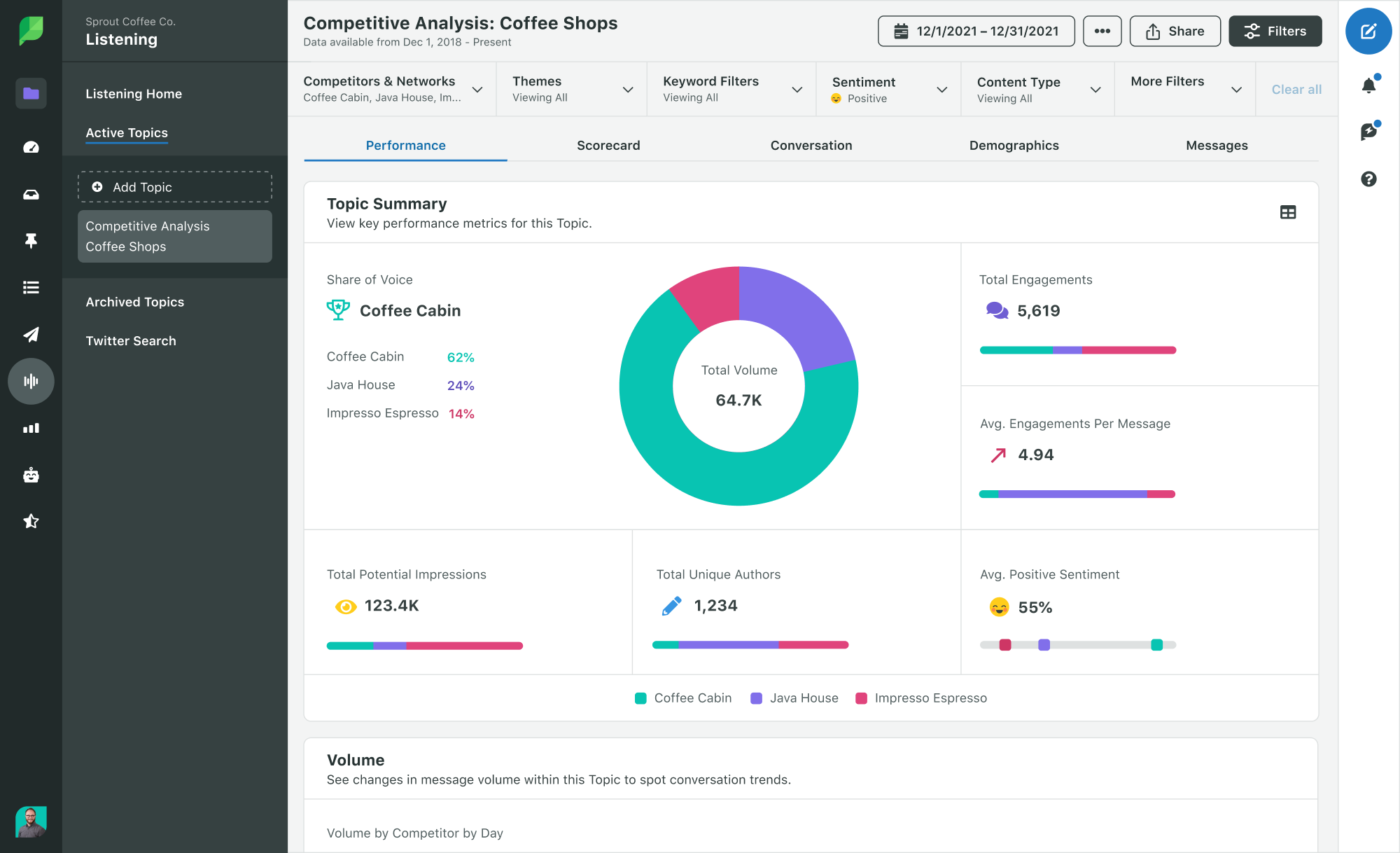
Create impactful posts using our Suggestions by AI Assist feature, enabled by AI prompts. Plus, use Sprout’s patented ViralPost technology to ensure your posts reach your intended audience at the most optimal times. The technology calculates your best times to publish content and enables you to automate or manually post them for maximum engagement and impact.
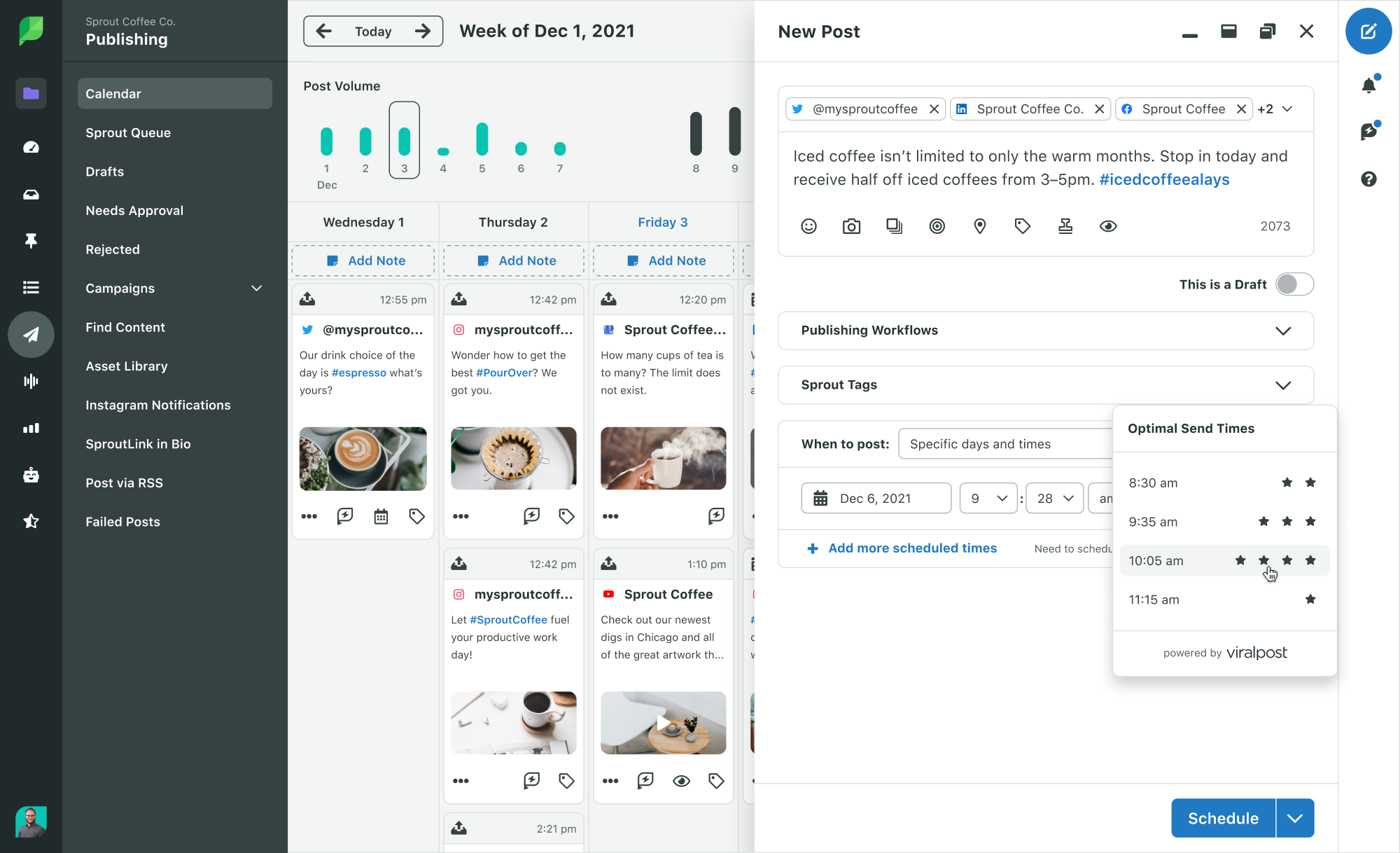
Sprout’s advanced AI-enabled Smart Inbox helps you quickly identify sentiment in incoming messages and review feeds. The posts are automatically assigned as positive, neutral or negative so customer care teams can prioritize messages accordingly and act swiftly.
Adopt these and other intuitive Sprout features to bring your data to life and transform your social and digital marketing strategies. Tie them all together using our intuitive report templates and share insights securely across teams within the organization for faster, smoother collaboration.
Sprout seamlessly fits into your existing tech stack without a manual setup so you’re quickly up and running. And since it’s powered by advanced machine learning, it continuously learns from your data, becoming smarter with time. Thus, alleviating the need for regular investment in upgrading your software.
2. Power BI
Power BI is a business analytics tool built for data analysis and insight visualization. It provides comprehensive brand insights by mapping millions of data points from different sources of varying complexities. Its especially useful if you’re proficient in Microsoft Excel as you can easily create and modify reports based on your requirements and share them with teams. You do need to have a coding background as you’ll need it to understand data analysis expressions (DAX) during data modeling and working with M code for data transformations.
3. MonkeyLearn
MonkeyLearn is a text analysis tool that uses AI for data analytics. It can be used for automating customer feedback and survey data analysis to identify topics relevant to your target audience and extract useful insights. Although aimed to help with customer experience management, the tool is not efficient in handling a high volume of customer care tickets, which makes it difficult to scale or integrate with customer support needs.
4. Tableau
Tableau is an analytics and data visualization platform you can use to understand your data for meaningful brand insights and to create reports in real-time. Like Sprout, Tableau offers robust connectivity across various data sources and offers a unified view of your data and insights. Its comprehensive capabilities require you to be trained on it so you can use the tool to its fullest potential.
5. Sisense
Sisense is an AI data analytics tool that can access multiple online data sources to provide insights in flexible reports. It can be used by teams who don’t have advanced technical expertise to conduct preliminary data analysis for business intelligence. The tool has a suite of advanced features as well but you’ll need to be trained on them before you can start using them for insights.
The future of AI in data analytics
AI analytics has mostly been used in critical industries and large enterprises such as healthcare and manufacturing to expedite processes, identify data fluctuations and get actionable insights. However, recent advancements in machine learning are poised to make it a standard practice across industries.
For one, AI tools have become more affordable and user-friendly. They are enabling brands to embrace innovation and face the competition more confidently. Companies can gather business intelligence from varied data sources easily for a deeper understanding of their market. Which in turn can be used to personalize customer interactions, enhance brand satisfaction and expand into new markets with foresight and precision.
Take advantage of AI-enabled automation to optimize and see the ROI of your social and digital data. Explore these cutting-edge AI marketing tools to propel your business forward by leveraging social data to win 2024 and beyond.
Share