How named entity recognition (NER) helps marketers discover brand insights
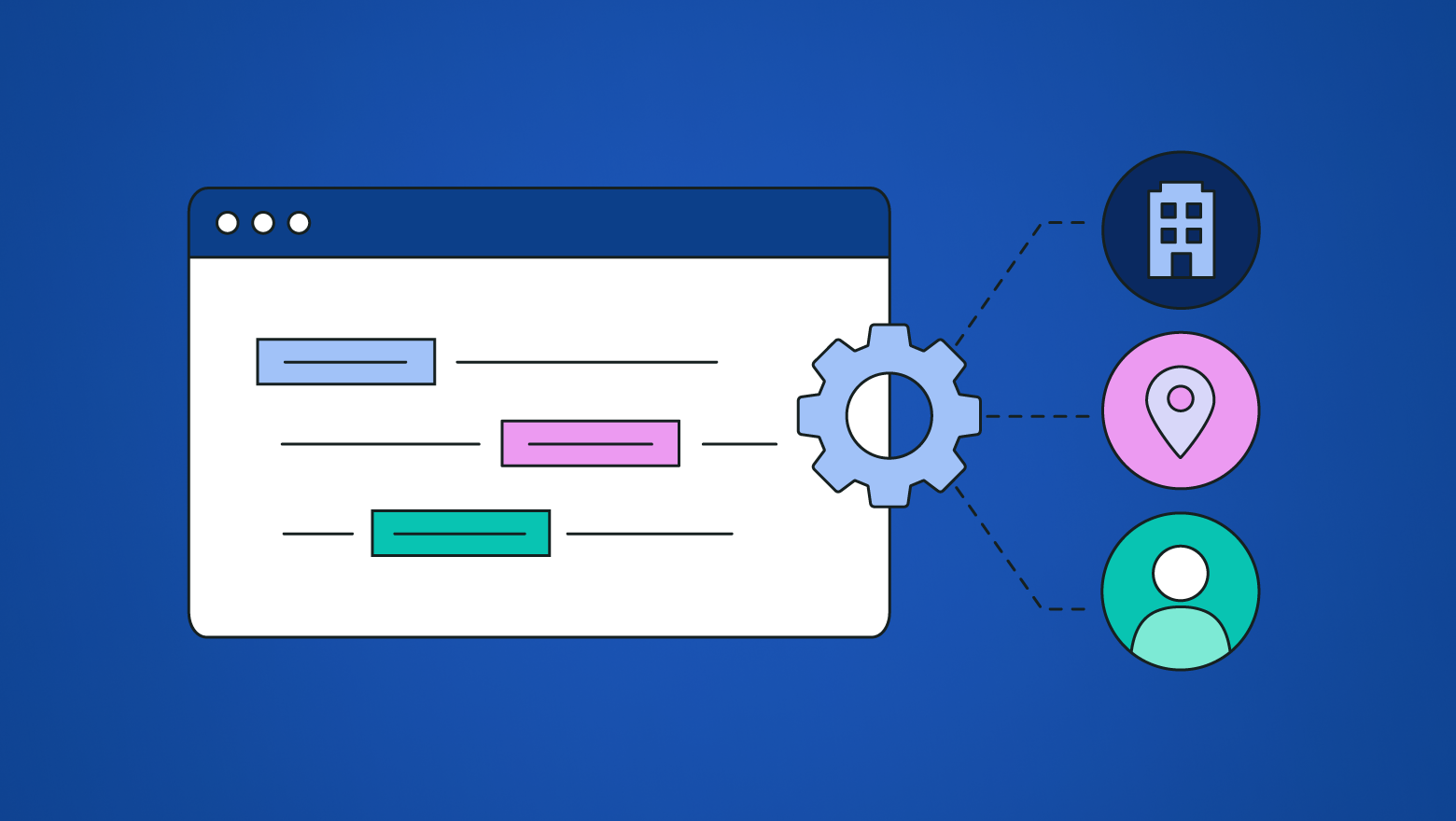
With trends emerging every day, social networks introducing new additions (hello, Threads!)—not to mention brand makeovers, like Twitter rebranding to X—marketing teams are forever playing catch up.
Staying agile seems daunting and finding meaningful insights from non-stop social and online chatter feels akin to finding a needle in a haystack. Add to that, tight budgets and limited manpower.
Fortunately, AI marketing techniques like sentiment analysis and machine learning (ML) enable marketers to overcome shrinking bandwidths and harness social listening for business intelligence. AI tools extract key data points from thousands of social conversations across multiple networks within minutes, giving you actionable insights that impact your market growth and revenue.
But how do these tools identify relevant information from the barrage of conflicting data online? How do they identify brand mentions for competitive analysis? And how do they distinguish between individuals, businesses or currencies in data?
Enter: named entity recognition (NER). This core AI technology works behind the scenes to power AI marketing tools, so you get critical, data-driven metrics from social and online data for strategic business decisions.
In this guide, we break down what NER is and how it benefits businesses. Plus, share a list of five tools with the best NER capability.
What is named entity recognition?
Named entity recognition is a subtask of artificial intelligence. It’s used in natural language processing (NLP) to identify and extract important information or “entities” in text. An entity can be a word or a series of words such as names of famous celebrities or cities as well as numerical data such as currencies, dates and percentages.
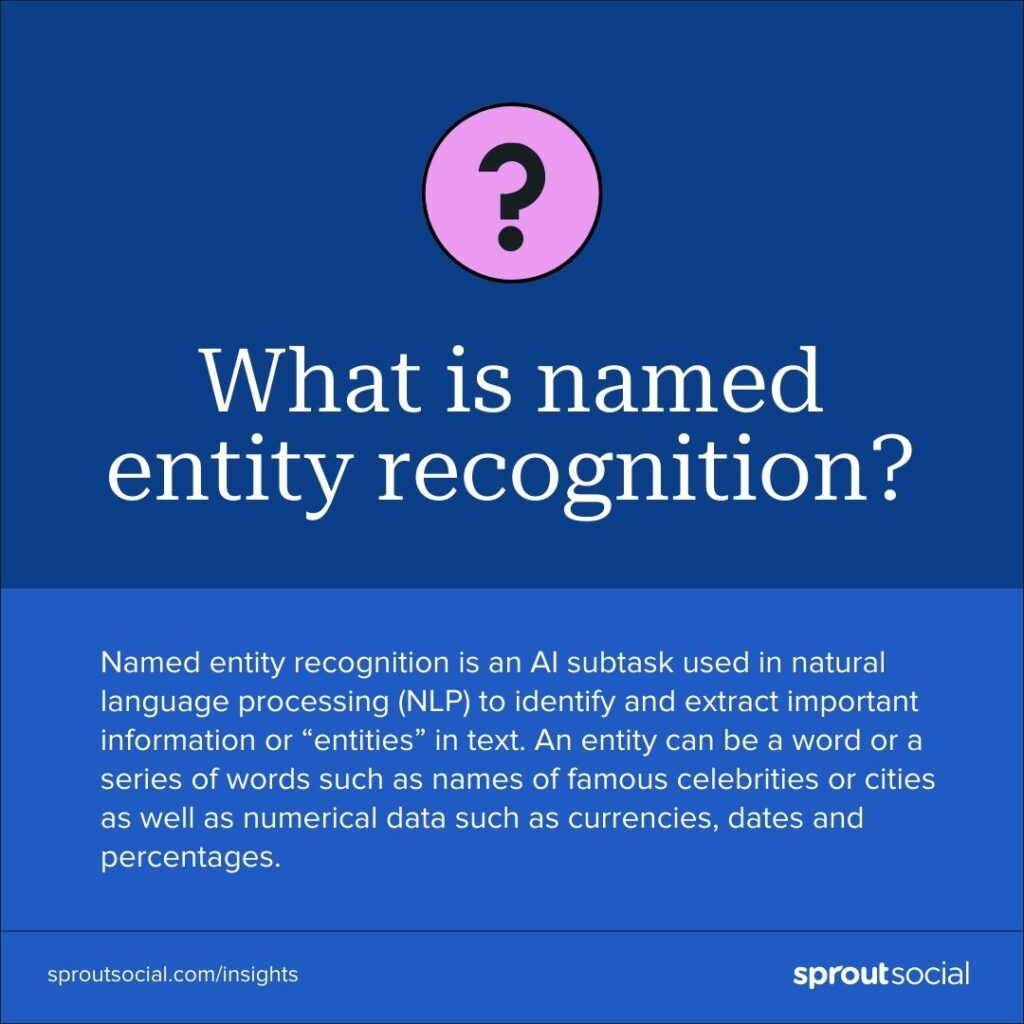
NER is used in AI marketing tools to automatically spot and categorize important information in data to conduct tasks like social listening, sentiment mining or brand analysis. NER is also crucial in search engines, enabling them to understand and recognize key elements in queries and then search and provide relevant results.
How does named entity recognition work?
Named entity recognition, or entity chunking, is an AI task that enables text analysis and assists in natural language generation (NLG)—a capability commonly used in chatbots, virtual agents and search engines.
NER is manually coded into a machine-learning model with annotated data to train the model into recognizing important entities from unstructured data. Manual tags are created so all similar NER entities are classified into a pre-determined category such as “people”, “locations” or “currencies”.
Misspellings and abbreviations are also encoded to assist in getting more accurate results. For example, the United States may be annotated as The United States of America, The US and U.S.
On average, an AI tool has upwards of 7 million NER entities. The more robust a tool’s NER, the more precise the results. It allows the tool to scan millions of data points in comments, social posts, reviews, news stories, etc. and immediately identify keywords for data analysis to reveal brand health or customer experience insights.
For example, in the sentence “Sprout Social, Inc. is ranked #2 on the Fortune Best Workplaces in Chicago™ 2023 SM List”, NER identifies and categorizes Sprout Social as a business, Fortune Best Workplaces as an award category, Chicago as a location in the US and 2023 as a calendar year.
In this way, tools powered by NER identify highly relevant entities from tons of scattered data to provide insights on competitors, customer demographics and emerging industry trends. These enable you to create data-driven, customer-centric marketing strategies that can improve your return on investment.
What are the business benefits of NER?
Many businesses are already using AI and ML for business intelligence. According to The 2023 State of Social Media Report, 96% of leaders agree AI and ML technologies are significantly improving business decisions, and 87% expect to increase AI and ML technology investments in the next three years.
Here is a breakdown of how NER is enabling this transformation.
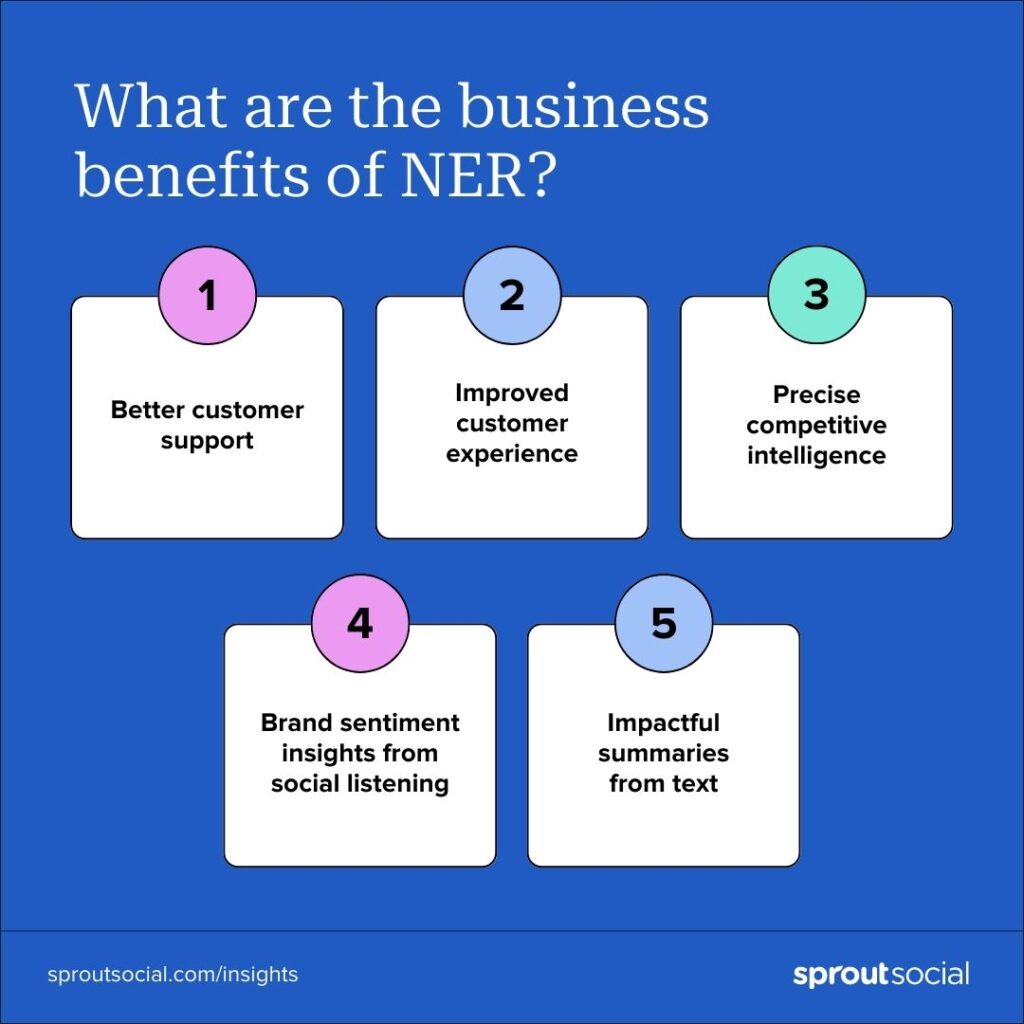
Better customer support
Per the same report, 93% of business leaders plan on increasing investments in AI tools to elevate customer support functions in the coming three years.
NER is pivotal in supercharging customer care functions. It helps an AI tool automatically categorize queries and complaints by identifying keywords (such as brand names or branch locations), so they’re queued and routed to relevant customer care teams for smoother support.
NER also enables marketing automation and assists in tailoring and optimizing customer care responses for maximum impact. For example, Sprout’s Suggested Replies helps support teams respond faster to commonly asked questions on Twitter. NER powers semantic analysis algorithms in the tool to understand messages contextually, identify topics and themes through keywords and then suggest the best-suited responses.
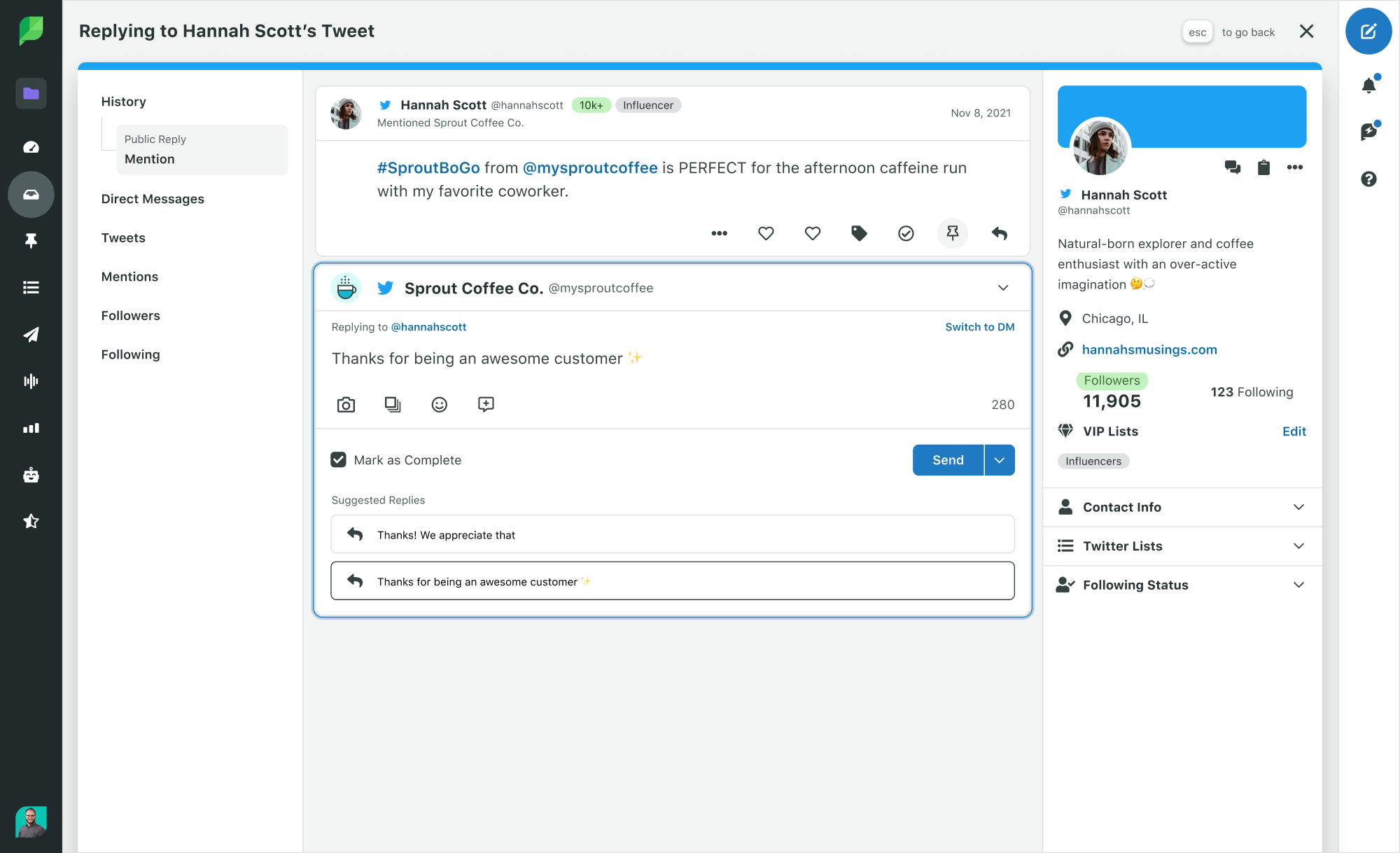
Improved customer experience
Named entity recognition also helps you find critical details in customer experience data to elevate customer delight throughout the purchase journey.
In Sprout, NER identifies and tracks keywords you define, including hashtags and @mentions, in a wide range of social listening sources like Reddit, Glassdoor and YouTube. Capture what customers are talking about and what their preferences are to identify how you can improve your brand.
These brand insights are also beneficial across the organization, informing targeted advertising, product enhancements and more engaging social content.
Precise competitive intelligence
NER algorithms identify and track competitors for competitive benchmarks and key performance indicators (KPIs) from customer and market data. For example, in Sprout, you’re able to track and analyze competing brands and their content simultaneously based on several KPIs like volume, type, frequency or hashtag usage with competitor reports and listening tools.
These insights provide a strategic guide to creating better brand experiences, from maintaining market share to tailoring your messaging for better audience engagement.
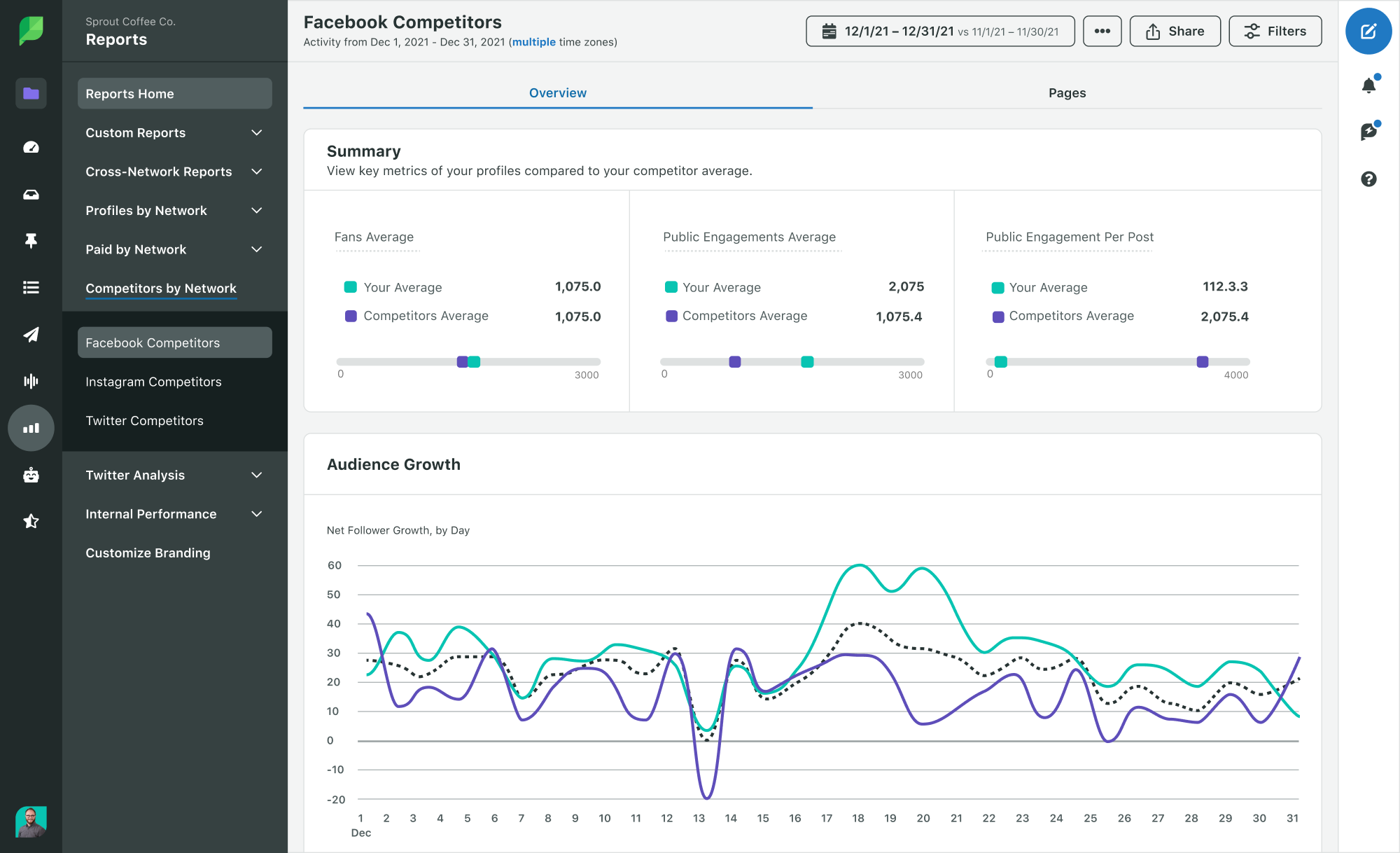
Brand sentiment insights from social listening
Forty-four percent of leaders agree one of the most important uses of AI and ML tools is understanding customer feedback in real time through sentiment analysis.
NER algorithms enable sentiment analysis in social listening data by extracting important entities from direct comments, brand mentions and other user-generated content. This enables you to measure what customers love about your brand and where to improve.
NER is also critical in tracking brand reputation. It helps AI tools identify negative brand mentions as and when they occur in social comments and DMs. This enables your team to be proactive and concentrate on taking relevant actions to resolve issues rather than spend time manually monitoring your brand health.
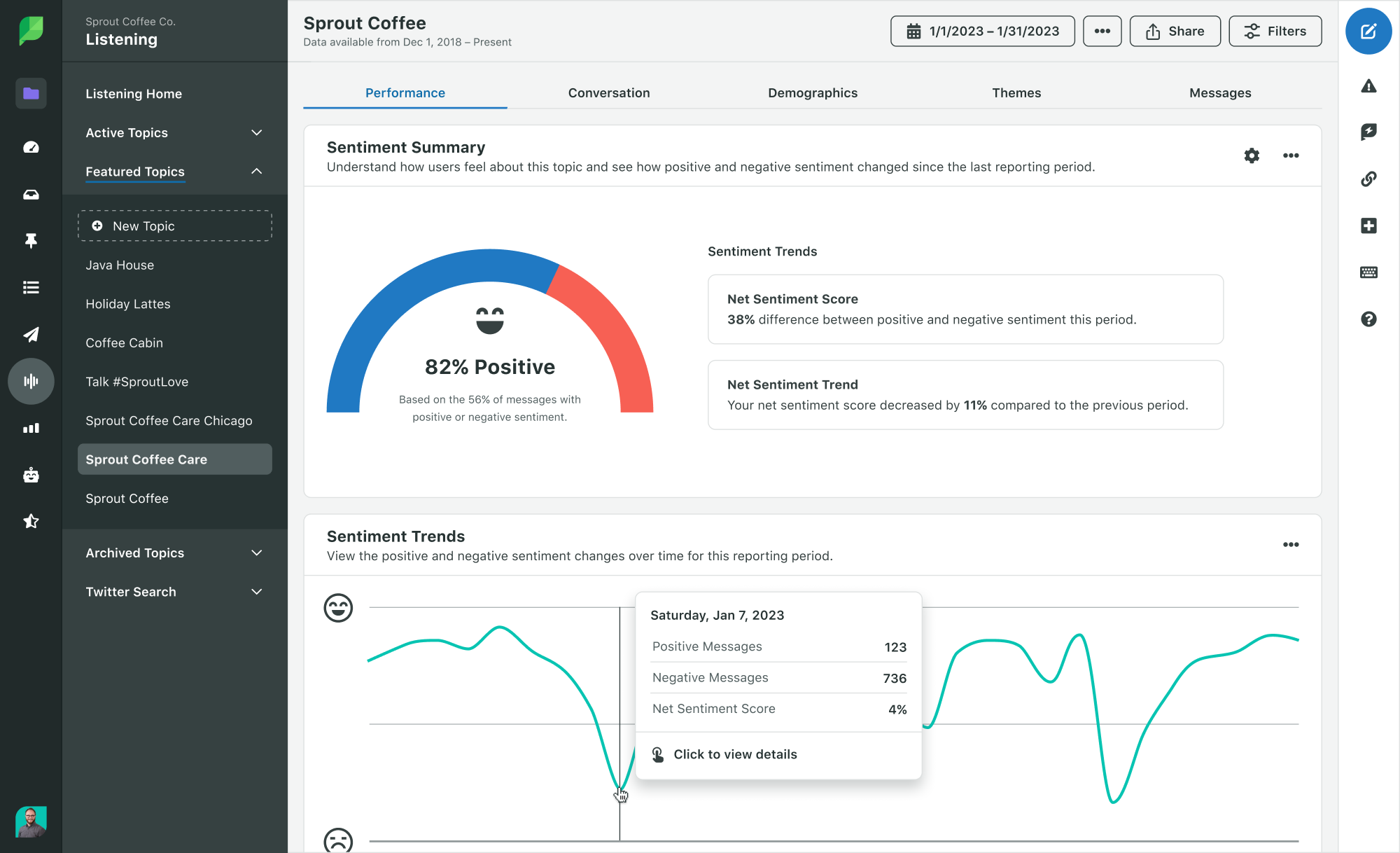
Impactful summaries from text
NER is widely used across industries to identify important entities in keywords, topics, aspects and themes in text sources to provide impactful summaries. These text sources include news articles, podcasts, legal documents, movie scripts, online books, financial statements, stock market data and even medical reports. NER plays an important role in how AI generative tools interpret queries or prompts. Click on the link to learn how to maximize the output from AI tools by using the best ways of writing AI prompts.
Summaries from these sources can serve strategic purposes such as brand reputation management, patient experience (PX) analysis or gauging a company’s financial performance over time.
How named entity recognition assists social listening
Social media listening can be overwhelming, especially if you have to manually search thousands of comments and posts for important brand and product insights on a regular basis.
AI-powered social listening tools, like Sprout, overcome this challenge by using technologies like NER. These algorithms automatically identify keywords in social chatter and discussions across social networks so AI tasks like sentiment analysis and machine learning can derive meaningful business insights from the listening data.
For example, Sprout’s Query Builder uses NER to keep a pulse on the social conversations happening around your brand. NER identifies and categorizes social listening data with keywords you‘ve pre-determined (brand names, product names, topics)—even misspelled names—behind the scenes.
Thus, it helps the Query Builder to sort through millions of data points and return only those messages that match your query. It also powers a spam filter to further refine the data.
Social listening can have many conflicting data points but entity chunking and semantic clustering overcome it by removing redundant data. This enables you to contextually see how often messages with a particular keyword are occurring. This is essential for customer support teams to identify common complaints in products and services.
Champion growth with NER-powered social listening
Marrying superior AI-driven brand intelligence capabilities with a user-friendly experience puts power directly in marketers’ hands. NER and social listening enable you to get insights in real time to stay ahead of competitors and deepen customer loyalty.
Use social listening to tap into the unfiltered thoughts of your audience and derive candid insights into your brand, products and services—and your competitors. Download this social listening cheatsheet to identify your listening goals and use social data to grow your entire business.
FAQs
What is the difference between NLP and NER?
NLP is an AI capability that analyzes human language rather than artificially developed ones such as computer coding. It enables an AI tool to contextually understand text data gathered from various digital sources like news articles, customer experience data, reviews, social media listening, etc.
NER is an AI task that identifies and extracts important information from text data to enable data analysis for brand and business insights.
- Categories
24 AI marketing tools your team should be using in 2025
Published on November 13, 2024 Reading time 12 minutes - Categories
AI in marketing examples and strategies you can use today
Published on October 15, 2024 Reading time 6 minutes - Categories
How to turn AI into your social media assistant when seasonality strikes
Published on October 3, 2024 Reading time 8 minutes
Share